Séminaire du Pôle Probabilités
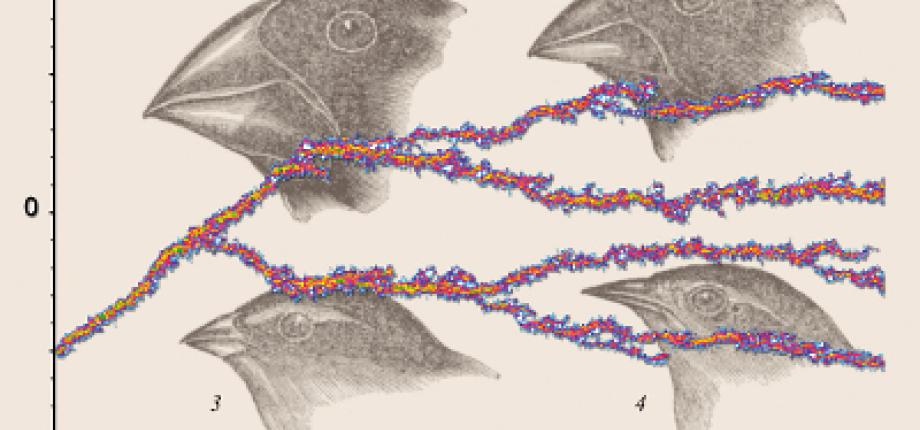
Lieu : nouvelle salle de conférence du CMAP (salle 3005, dernier étage, aile 05 - soit le long couloir perpendiculaire à l’aile 0)
Horaire : un mardi sur deux, de 10h à 11h
Remarque : aux mêmes dates et dans la même salle, à 11h30, a lieu le séminaire du pôle Analyse.
Les organisateurs : Quentin Cormier, Stefano De Marco, Fabrice Djete, Clément Foucart, Cyril Marzouk & Milica Tomasevic
(à contacter à l'alias orga-seminaire-pole-proba "at" meslistes.polytechnique.fr)
Novembre 2024
Mardi 19 Novembre
Marek Kimmel (Rice University, Houston, Etats-Unis) - Estimating Past Events in Cancer Through Stochastic Modeling of DNA Sequencing Data
The starting point of stochastic modeling of cancer is branching evolution, in which cells share similar growth and mutation rates (the clonal phase). Later, some cells acquire mutations in their DNA that may grant a selective advantage to the subclones initiated by these cells (they grow faster or die slower), so that the tumor is now composed of distinct clusters of cells. We discuss briefly mathematical and computational approaches to such modeling, based on models of population genetics (such as the coalescent of Griffiths-Tavaré) and branching processes (Lambert and Durrett’s approach).
The inference task is estimation of evolutionary parameters based on one snapshot in time (DNA sample from one time point). The emphasis is on arrival times of sub-clones and their growth rates with mutation rates variability confounding the estimates. We derived estimating equations, which can be solved and, although simplified, allow insights into estimability. We illustrate the considerations with simulated and data-based examples, which link outcomes of such estimations to screening for early cancer detection and resulting decision-making.
One of the great hopes of cancer research is single-cell DNA sequencing which, in theory, allows direct visualization of the pedigrees of clones in a tumor. For various reason, this promise is still awaiting fulfillment. One of the difficulties is resolving clones initiated by advantageous mutants from those arising from random fluctuations, as in the “jackpot” effect, observed already in 1940’s by Luria and Delbrűck. We developed a method based on Tibshirani clustering that seems to be resulting in less misclassification than some others. Results will be presented.
Another important aspect is that human cancers frequently have prominent spatial structure. One example is urinary bladder cancer. The underlying organ is a roughly spherical ball. Based on a unique collection of whole organ specimens, we can obtain insight into order and timing of mutations in this specific case, using a parsimonious approach based on branching processes with immigration model.
Collaborators (order idiosyncratic): Andrew Koval, Emmanuel Asante, Ren-Yi Wang (Rice U.), Khanh Ngoc Dinh and Simon Tavaré (Columbia U.), Bogdan Czerniak and Peng Wei (MD Anderson), Roman Jaksik, Monika Kurpas, and Pawel Kuś (Silesian Tech.), Olga Gorlova and Ivan Gorlov (Baylor College of Medicine).
Décembre 2024
Mardi 03 décembre
Mehdi Talbi (Univ. Paris Cité) -
Mardi 17 décembre
Paul Gassiat (Paris Dauphine) -
Janvier 2025
Mardi 07 janvier
Mardi 21 janvier
Février 2025
Mardi 04 février
Mardi 18 février
Mars 2025
Mardi 04 mars
Mardi 18 mars
Avril 2025
Mardi 1 avril
Mardi 29 avril
Mai 2025
Mardi 13 mai
Mardi 27 mai
Juin 2025
Mardi 10 juin
Mardi 24 juin
____________________________________________________________
Anciens séminaires
Novembre 2024
Mardi 5 Novembre
Arno Siri-Jégousse (UNAM Mexique) - Évolution et généalogies de populations autosimilaires
Octobre 2024
Mardi 22 Octobre
Michael Goldman (CMAP)- Recent progress on the optimal matching problem
In this talk I will review some recent progress in the understanding of the (random) optimal matching problem. While the work of Ajtai-Komlos-Tusnady in the 80's on this classical optimization problem attracted a lot of attention from the probability community (see the book by Talagrand), this problem has seen a renewed interest from the PDE community thanks to the ansatz proposed by Caracciolo Lucibello, Parisi and Sicuro in 2014. I will explain to which extent this ansatz can be rigorously justified and show how it leads to a deeper understanding of this problem.